DataOps and the evolution of data analytics
Data operations is considered a game-changer in data analytics
Add bookmark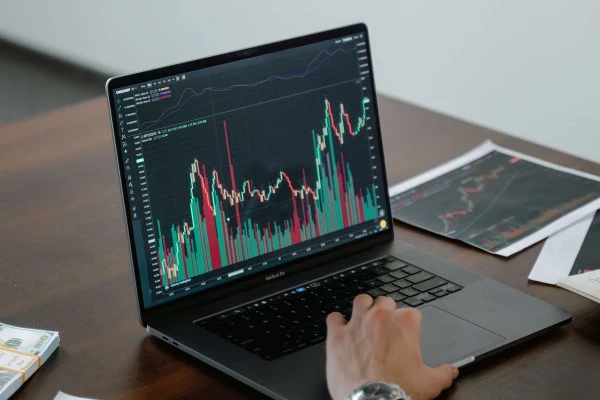
Data operations (DataOps) refers to practices, processes and technologies that combine an integrated and process-oriented perspective on data with automation and methods from agile software engineering. It aims to promote continuous improvement in data analytics to drive optimization and improvement.
The goal of DataOps is to deliver value by creating predictable delivery and change management of data, data models and related artifacts, automating the design, deployment and management of data delivery with appropriate levels of governance, according to Gartner. The global DataOps platform market is projected to reach US $10.9 billion by 2028.
DataOps is considered an evolution of data analytics designed to create greater business value from big data, combining DevOps, Agile, Lean and Total Quality Management (TQM) methodologies to manage data in alignment with business goals.
Agile processes are used for data governance and analytics development while DevOps processes are used to optimize code, product builds and delivery. As with Lean, DataOps requires a clear focus on the customer and the creation of simple, standardized and automated processes that eliminate waste, redundancy and cost. Like TQM, DataOps adopts continuous testing, monitoring and benchmarking to detect issues before they turn into major problems. All such elements embrace a culture of continuous business improvement.
DataOps can be applied to any business process or analytic solution that involves extracting, ingesting, cleaning, moving, storing, transforming, integrating or aggregating data. Signs that your business could benefit from Data Ops include:
- Your data team is flooded with minor request tickets and is burning out.
- Users don’t trust data because it contains too many errors.
- You are too busy to implement predictive analytics.
- Source system changes keep breaking your data integration jobs and data pipelines.
- You have difficulty meeting service level agreements (SLAs) for critical applications.
- Data analysts recreate existing data pipelines with minor variations.
A game-changer in data analytics
“Think of DataOps as the behind-the-scenes crew that helps data teams work better and faster,” Conor Twomey, head of customer Success at KX, tells PEX Network. It is all about making sure everyone involved in handling data, from the tech folks to the analysts, can collaborate smoothly – much like what DevOps did for software development but for data workflows, he adds.
“DataOps is a game-changer in data analytics. It’s like having a pit crew for your data, ensuring everything runs at top speed with as few hiccups as possible. This way, companies can get from raw data to insights that help make smart decisions quickly and reliably, which is super important for organizations to thrive in this fast-paced world.”
DataOps is a broad concept in the sense that it ties into a wide range of different capabilities and can also depend on what the organization needs from that individual, says Luc Simpson-Kent, data science managing consultant at Harnham, a global data recruitment company. “For instance, you might have a DataOps person who is more focused on the data development side or find those more focused on operations, for example.”
Benefits of DataOps
Teamwork and delivery speed
DataOps can benefit organizations by improving teamwork and delivery speed, Twomey says. “It’s all about getting folks from different departments to play nice and work together on data projects. It will certainly help you get your data projects done quicker so you can make fast, informed decisions.”
Trust and growth
Another benefit is its ability to facilitate trust and growth, Twomey adds. “Organizations can trust its data more because DataOps keeps a tight check on quality, and it’s built to handle more data without getting a headache.”
Collaboration and efficiency
DataOps professionals are people who are agile and interactive in terms of development – exploring and curating new ways of responding to development cycles. “This can help and improve collaboration across teams,” says Simpson-Kent. In essence, it’s about shortening the timescale on delivering insights and ensuring that the factory or infrastructure is ready for others to use it. “Data within an organization can be scattered far and wide so a DataOps professional is there to ensure that the data is streamlined properly and is ready for the next process.”
Automated efficiency
“Automation, of course is linked to efficiency,” Simpson-Kent adds. By repeating tasks and constantly improving efficiency, DataOps professionals will ultimately reduce errors and therefore speed up and improve processes.
Challenges of DataOps
Shifting mindsets
On the other hand, DataOps can have some downsides, says Twomey. “It’s essentially adopting a new playbook and shifting to a DataOps mindset can be tough.” It’s a new way of working that not everyone will be ready for. “In addition, you need people who know their stuff across different areas, and talent isn't always easy to find.”
Complex technology
Another DataOps challenge is the technology required, Twomey says. “Hooking up all of the different data tools can get tricky and, as things become more automated, making sure you're still following the rules can get complicated too.”
Cost of implementation
Cost is another key consideration of DataOps, says Simpson-Kent. “Not only does it pose a challenge in that businesses need to invest money in hiring talent with the correct skillsets, but also in integrating systems necessary to the practice. However, while there may be an initial implementation cost, there are also opportunities for cost savings via improving a business’ capabilities and data governance process.”
Energy use
DataOps processes can be very energy intensive. AI technology is seen as one potential source for helping to reduce DataOps emissions by using fewer resources, but organizations should not deceive themselves that AI is currently a green technology for DataOps emissions.
DataOps in healthcare - case study
DataOps has demonstrated significant potential in driving innovation and optimization in healthcare, writes Mark A. Johnston, VP, global healthcare and life sciences innovation and strategy at InfoVision. A 2023 HIMSS Analytics study revealed that hospitals embracing DataOps witnessed an average 18.5 percent improvement in operational efficiency. A 2022 report by the Healthcare Information and Management Systems Society (HIMSS) revealed a 15 percent reduction in medication administration errors within organizations adopting DataOps principles.
Johnston cites the example of Boston Children’s Hospital, which faced fragmented data systems across departments impeding research efforts and hindering effective clinical decision-making. By adopting DataOps, the hospital implemented a cloud-based data lake and automated data pipelines to integrate and clean data, resulting in a single source of truth. This reduced research lead time by 40 percent, improved medication adherence by 12 percent and enabled faster diagnosis and treatment for rare diseases.
READ: PEX Report 2024: Global state of process excellence. Learn how companies are capitalizing on innovation in a dynamic business environment, with success stories from the likes of Johnson & Johnson, Swiss Re and Bosch Group.