The convergence of BPM & AI: Enabling real-time process optimization
The evolving nature of emerging artificial intelligence (AI) is having a transformative impact on business process management (BPM)
Add bookmarkListen to this content
Audio conversion provided by OpenAI
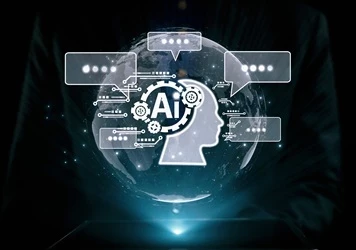
For decades, business process management (BPM) has been a stalwart of process and operational excellence (OPEX). It still is today, but the evolving nature of emerging artificial intelligence (AI) is having a transformative impact on the BPM landscape. From opening up vast new opportunities to creating complex new challenges and considerations, AI technology is playing a key role in shaping the future of BPM.
Vinod Srinivasa, assistant VP of OPEX at America’s leading multinational bank, will be speaking at All Access: Future of BPM, exploring how to build a forward-thinking BPM strategy and drive new opportunities in operational efficiency.
Ahead of the event, we sat down with Srinivasa to get his insight on the evolution of BPM amid the growth of advancing AI technology.
Don't miss any news, updates or insider tips from PEX Network by getting them delivered to your inbox. Sign up to our newsletter and join our community of experts.
PEX Network: How is modern BPM converging with a new generation of AI technology?
Vinod Srinivasa: While BPM traditionally focuses on streamlining and automating business workflows through structured processes, AI introduces advanced data analytics, machine learning and cognitive capabilities to further enhance these workflows, making them smarter, more adaptive and capable of real-time optimization.
This convergence is driving significant advancements in operational efficiency, customer experience and decision-making across industries.
Here are a few of the significant convergence outcomes along with industry use-cases:
- Real-time decision-making and process agility: AI’s integration into BPM enables real-time decision-making, which is crucial for businesses operating in dynamic environments. BPM systems use AI to analyze vast amounts of data in real-time, predict future outcomes, and recommend actions, helping businesses adapt their processes immediately based on emerging trends or customer needs. For example, Siemens uses AI to improve its manufacturing processes by integrating predictive analytics with BPM. Using machine learning algorithms, Siemens is able to forecast equipment failures before they occurred, allowing for proactive maintenance that minimized downtime. This predictive capability was integrated into the BPM workflow, allowing the system to trigger maintenance alerts and reallocate resources to ensure uninterrupted production.
- Going beyond rule-based automation: Traditionally, BPM was associated with automation of predefined, repetitive tasks. However, with the integration of AI technologies like natural language processing (NLP) and cognitive computing, intelligent process automation has emerged as a game-changer. AI enables BPM systems to automate not just rule-based tasks but also more complex, dynamic processes that require decision-making based on unstructured data.
- Customer experience (CX) enhancement through AI-driven BPM: CX is an area where the convergence of BPM and AI is making a big impact. Traditional BPM focused on improving process efficiency but did not account for the need to personalize interactions at scale. AI introduces capabilities like personalization, sentiment analysis and chatbots, which can be integrated with BPM to create more personalized, responsive and proactive customer service processes. For instance, HSBC has implemented an AI-powered BPM solution that combines chatbots for customer service automation and machine learning algorithms to predict and respond to customer inquiries based on historical data. As a result, HSBC can improve customer satisfaction by providing faster, more relevant responses to inquiries, while its BPM system ensures the seamless routing of more complex issues to human agents.
- Process mining with continuous process monitoring and optimization: Another powerful aspect of AI in BPM is process mining, where AI is used to continuously monitor, analyze and optimize business processes. Process mining allows businesses to visualize and understand their existing workflows, identify inefficiencies and make real-time adjustments to improve performance.
- Enhanced predictive and prescriptive analytics: AI’s ability to provide predictive and prescriptive analytics is also enhancing BPM. Traditional BPM focuses on process control and optimization, but with AI, businesses can forecast future trends and prescriptively recommend actions based on predictive insights. For instance, Amazon uses AI-driven BPM to optimize its supply chain processes. By applying predictive analytics, any e-commerce firm can anticipate product demand across different regions and adjust its inventory and delivery workflows accordingly. If an AI model predicts a surge in demand for a particular product, the BPM system automatically triggers the necessary steps – reordering stock, redistributing inventory or rerouting shipments – to meet demand without delay.
PEX Network: What process enhancements can businesses gain by combining BPM with AI?
VS: By combining BPM’s structured approach to process optimization with AI’s ability to analyze large datasets, learn from patterns and make autonomous decisions, firms can achieve significant process enhancements including greater operational efficiency, improved customer experiences, risk reduction and cost savings.
In the banking, financial services and insurance (BFSI) industry, this convergence brings key benefits including:
- Increased operational efficiency through automation: BPM is largely about automating workflows to eliminate inefficiencies and ensure compliance. When combined with AI, businesses can elevate this automation from simple, rule-based tasks to more complex, decision-based actions that improve operational efficiency. For example, Standard Chartered Bank integrated AI with its BPM system to automate the customer onboarding process. By leveraging AI-driven document processing, the bank was able to extract and analyze customer data from forms and documents using NLP and optical character recognition (OCR), automatically populating account details into their BPM system. This reduced the need for human intervention, minimized errors and accelerated the onboarding process.
- Enhanced risk management and compliance: In BFSI, compliance with regulations like Know Your Customer (KYC) and Anti-Money Laundering (AML) is crucial. AI enhances BPM systems by automating risk assessments and compliance checks in real-time, enabling faster responses to potential issues and improving accuracy in reporting. For instance, JPMorgan Chase has adopted AI and BPM technologies to enhance its AML compliance processes where AI models are used to scan and analyze large volumes of transaction data, detecting patterns and flagging suspicious activities. These insights are seamlessly integrated into the BPM system, where workflows are automatically triggered to escalate the flagged cases for further investigation. This not only speeds up compliance efforts but also minimizes human error and reduces the chances of regulatory fines.
- Superior CX through personalization: BFSI customers increasingly expect personalized services, tailored to their specific needs and preferences. Combining BPM with AI enables financial institutions to deliver highly customized experiences, especially in areas such as product recommendations, customer support, and financial advice. Bank of America’s Merrill Lynch platform leverages AI and BPM to deliver personalized financial advice. The AI engine analyses customer data, including transaction history, risk profiles, and spending patterns, to generate tailored investment strategies and product recommendations. BPM is used to ensure that these recommendations are delivered to the right customers at the right time, through the most appropriate channels. As a result, Bank of America can offer a more personalized experience while maintaining operational efficiency.
- Improved fraud detection and prevention: Fraud detection in BFSI has traditionally been dependent on manually identifying patterns and anomalies. However, with AI, BPM systems can continuously analyze transaction data and detect fraudulent activity in real-time, enhancing fraud prevention efforts. For example, American Express has integrated AI with BPM to enhance fraud detection across its global cardholder base. The AI model monitors transactions in real-time, learning from historical data to detect unusual behavior such as irregular spending patterns or geographical anomalies. Once the AI flags potential fraud, the BPM system automatically triggers a workflow to freeze the account, notify the customer and initiate an investigation.
- Increased productivity and cost savings: By automating routine and complex tasks, AI combined with BPM can significantly reduce the time spent on manual processes, thus driving productivity. Employees are freed-up to focus on higher-value activities, while the BPM system ensures that tasks are executed consistently and efficiently. For instance, Allianz, a global insurance provider, uses AI-powered BPM systems to automate claims processing. The AI analyzes submitted claims, cross-references data with existing policies and provides initial recommendations about the validity of the claims. BPM ensures that these claims are routed through the correct approval processes without human intervention. This reduces the time spent on processing claims and increases customer satisfaction by speeding up claim resolution.
PEX Network: What are the biggest challenges of combining BPM with evolving AI?
VS: The integration of BPM with AI offers transformative benefits for businesses. However, the convergence of these two technologies is not without its challenges. While AI promises to elevate BPM systems, the combination also brings its own set of technical, organizational and strategic hurdles. Below are some of the major challenges businesses face when combining BPM with emerging AI, along with insights on how organizations are navigating these issues.
- Data quality and integration: Ensuring that data from disparate sources within an organization is seamlessly integrated into both the AI and BPM systems can be difficult. Inaccurate or incomplete data can result in AI models making incorrect decisions, leading to flawed process automation and potential operational inefficiencies.
- Complexity of AI model development and maintenance: Integrating AI with BPM requires developing sophisticated machine learning models that can continuously learn and improve over time. This requires specialized expertise in AI and deep learning, which is not always readily available within an organization. In addition, maintaining and updating these models to ensure their continued relevance and effectiveness is a significant challenge.
- Resistance to change: Integrating AI into BPM often requires a shift in mindset – from manual, human-driven decision-making to AI-augmented, data-driven processes. Employees may feel threatened by these changes or may not fully trust the AI’s ability to make accurate decisions. This resistance can hinder the successful implementation and adoption of the new system.
- Security and ethical concerns: As AI becomes more integrated with BPM, concerns around data privacy, security and ethical decision-making arise. For example, when AI is used to process sensitive data, such as customer financial information or personal health records, there are significant risks related to data breaches and unauthorized access. Moreover, AI models can sometimes generate biased outcomes, which may lead to unethical or discriminatory decisions.
- Scalability and flexibility of integration: Scaling AI-powered BPM systems across the entire organization or adapting them to different business units can be a significant challenge. While BPM systems may be effective for specific, well-defined processes, integrating AI may require a complete overhaul of existing systems or significant modifications to align with AI capabilities.
PEX Network: How can businesses get optimal results through the convergence of BPM and AI?
VS: To ensure top-notch results from the convergence of BPM and AI, organizations should focus on:
- Aligning AI and BPM goals with business objectives
- Ensuring data quality and integration
- Refining AI models continuously
- Using process mining for optimization
- Fostering cross-functional collaboration
- Adopting Agile methodologies
- Implementing human-AI collaboration
- Actively monitoring performance
By taking a comprehensive, strategic approach to combining BPM and AI, businesses can unlock the full potential of these technologies – improving operational efficiency, customer satisfaction and decision-making across the organization. The result will be a dynamic, adaptive and data-driven business that is better equipped to thrive and lead in the digital age.
Learn more about the BPM landscape of tomorrow at All Access: Future of BPM. Register for free now!
PEX Report 2024 global state of process excellence
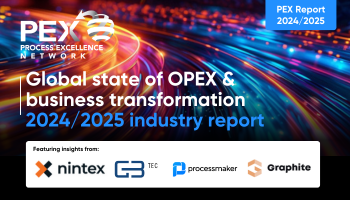
PEX Network's annual report reveals the current state and future trends of process excellence. Based on a study profiling hundreds of global process excellence leaders, the report provides insights on how to leverage innovation, generative AI, and process intelligence to achieve operational excellence, customer-centricity, and organizational growth. Download your copy of the PEX Report 2024/2025 today and benchmark your process excellence journey against your peers.
Download Now