6 steps to reducing process debt for long term OPEX
Process debt is the sum of inefficiencies, risks and compliance failures that accumulate as processes deviate over time
Add bookmarkListen to this content
Audio conversion provided by OpenAI
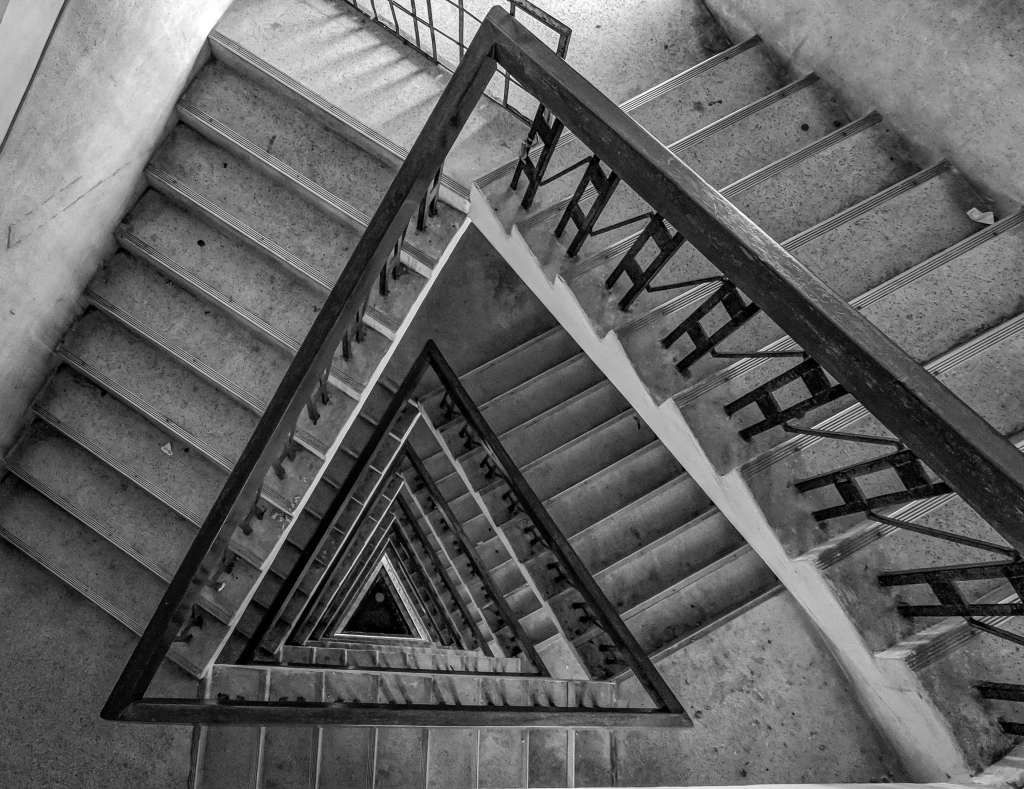
We’ve entered The Great Recalibration – a defining era where organizations are responding to economic uncertainty, shifting workforce dynamics and the accelerated adoption of artificial intelligence (AI).
Unlike the reactive churn of The Great Resignation or The Big Quit, this moment is about strategic refinement. Businesses are optimizing operations, rethinking cost structures and realigning technology investments to build resilience for the long haul.
In this landscape, process mining and process intelligence have emerged as mission-critical tools, empowering leaders with the data-driven visibility needed to confidently navigate complexity and change. Yet, amid this transformation, a silent threat looms: process debt.
Often overlooked, process debt is the hidden accumulation of inefficiencies – fueled by outdated assumptions, manual workarounds and fragmented decision-making. Much like technical debt in software, it represents the widening gap between how processes are designed to work and how they actually run. Left unaddressed, it weakens compliance, drains resources and quietly undermines transformation efforts.
Let’s dig into what process debt is, how to identify it and establish a proven, step-by-step framework for reducing it using process intelligence and process mining.
Process debt is like shadow IT
Process debt is the sum of inefficiencies, risks and compliance failures that accumulate as processes deviate from their intended design over time. It stems from:
- Inconsistent process execution
- Undocumented workarounds
- Missing or ineffective controls
- Lack of visibility into performance or risk trends
- Reactive firefighting instead of proactive improvement
Symptoms of process debt include:
- High rework rates and bottlenecks
- Low automation rates and excessive manual handling
- SLA breaches and customer complaints
- Unpredictable costs and audit findings
- Fragmented or overly complex process variants
How to reduce process debt: A step-by-step guide
Step 1: Establish your baseline with process and task discovery
Goal: Reveal the true state of your processes and user interactions, based on data – not assumptions.
Action: Use process discovery and mapping to generate a realistic view of end-to-end process directly from system logs (e.g., SAP, Salesforce, ServiceNow). Common inefficiencies and complexities range from a lack of process definition, ownership and understanding to ineffective performance measurement, high turnover, poor design/execution and unaligned business value, to list just a few.
Don’t stop at the system level. Incorporate task mining to capture what happens on the desktop between system events – those critical human-in-the-loop actions often invisible in event logs.
Outcome: This dual-lens approach surfaces not only the structural issues but also the micro-frictions that contribute to process debt—like excessive application switching, manual workarounds, rework loops, and inefficient copy-paste behaviors.
Step 2: Identify high-risk process debt areas
Goal: Prioritize areas where debt has the highest business impact.
Action: Leverage process mining to quantify:
- Violation rates of business rules or controls
- Volume and cost of rework or manual steps
- Variants outside standard operating procedures
Combine this with task-level insights to expose why certain variants exist – such as agents bypassing systems to expedite processes, often introducing compliance risk. For example, a health insurer used Apromore to identify that claims with excessive processing time correlated directly with users opening outdated policy PDFs rather than system records, detected only through task mining.
Outcome: A ranked list of “debt hotspots” with measurable key performance indicators (KPIs) such as percentage of service level agreement (SLA) breaches, time/cost per case and automation potential can help inform improvement plans, focusing on where to invest, change or automate for the highest business impact.
Step 3: Run “what-if” simulations before you change
Goal: Test the return on investment (ROI) and compliance impact of changes – without risk.
Action: Use advanced process simulation and modeling to test the impact of proposed changes like removing a manual approval, adding a control or automating a task. This includes task automation simulation powered by insights from task mining. What-if simulations empower users to forecast the impact of changes on key metrics like lead time, resource load or compliance rates.
Outcome: Data-driven confidence in decision-making, reduced trial-and-error and clear justification for automation or redesign investments.
Step 4: Monitor and predict compliance in real-time
Goal: Prevent future process debt from forming.
Action: Continuously test key controls and get alerts when risks arise – before they lead to violations or fines! Rule-based task mining enables compliance controls to be visible and enforceable at the task level
Outcome: A predictive compliance posture, with early warnings and trend analysis built into your day-to-day operations.
Step 5: Use root-cause analysis to drive strategic fixes
Goal: Eliminate recurring problems at the source.
Action: Deploy AI-powered root-cause analysis to trace the origins of bottlenecks, SLA violations or deviations. Understand why they occur – not just where!
Outcome: Targeted interventions that go beyond surface-level fixes and address the root issues causes of process debt.
Step 6: Enable continuous improvement loops
Goal: Institutionalize a proactive, adaptive process culture.
Action: Use Process KPIs and dashboards to track improvements, measure gains and spot new issues. Align with Agile or OKR-based governance for rapid iteration.
Outcome: A living, breathing process excellence model where improvement becomes business-as-usual.
Combining process mining, task mining and digital twin simulation enables a true “round trip” to process excellence. This iterative journey starts by mapping and analyzing the current state, uncovering inefficiencies through task-level insights, simulating improvement scenarios and implementing and monitoring changes in real time. This continuous feedback loop – fueled by data and AI – helps to identify and therefore eliminate process debt to drive sustained gains in performance, compliance and operational agility.
Real-world value: What’s at stake?
Organizations that reduce process debt can not only significantly reduce process-related risks but can also drive true business value.
A Fortune 500 insurance provider partnered with Apromore to transform its auto claims processing, addressing challenges like inefficient manual processes, lack of transparency and compliance risks. This led to delays that negatively impacted customer experience and regulatory adherence.
With Apromore’s process mining platform, the provider gained visibility into the claims process, identifying bottlenecks and inefficiencies, and as a result, reduced claims processing times by 39 percent through the elimination of rework and automating critical steps of claims processing. Furthermore, real-time control testing ensured claims were handled according to industry standards, reducing violations by 46 percent.
Much like costly technical debt or shadow IT, process debt is an invisible cost until it manifests as customer dissatisfaction, a compliance breach and fine or operational drag. It can be managed by recognizing it as such – formalizing it, prioritizing its resolution and using relevant metrics to keep track of its progress. By combining process mining, simulation, compliance monitoring and task mining in a continuous optimization plan through process intelligence, organizations can reduce process debt in a measurable, strategic way.