How process mining analysis can improve customer experiences in banking
Process mining contributes to customer experience improvement
Add bookmark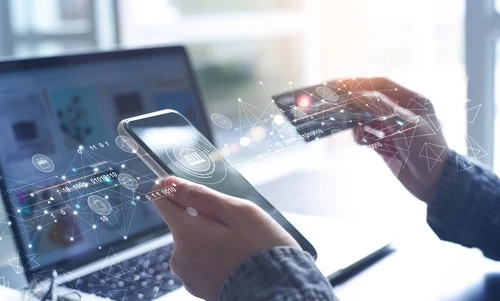
Process mining analyses should link directly to strategic goals. When used in concert with a transformation suite, we can achieve this. One common strategic goal in banking is improving the customer experience (CX) of banking services.
Customers now expect an Amazon-like experience when managing their finances, and to deliver this level of experience, banks need to identify where their processes are falling short. In this article, you’ll get a specific approach on how to enhance a process mining analysis to address this customer experience challenge.
Watch the on-demand webinar Fearlessly Dominate the Digital Banking Revolution with Process Mining to learn how to master the digital banking revolution.
Process selection and data collection
It’s not difficult to identify a process that’s a critical, customer-facing process. Account opening, security verification or incident resolution are all typical, banking-specific functions that can make-or-break how customers perceive the institution.
Once selected, immediately engage CX teams (or the team who owns this function). Most banks already run taskforces who have done excellent work mapping customer journeys and identifying pain points and will have collated the critical touchpoints that determine whether an experience is judged ‘good’ or ‘bad’ via direct feedback.
Image: Customer journey mapping in ARIS
In CX language, these inflection points are called ‘moments of truth’ where banks have a chance to impress (or distress) customers. As process miners, we want to understand how to incorporate these touchpoints into our process dataset.
Too often, process mining projects are fixated on system data, which, of course, is where we must start, but certainly not end. However, in addition to process event data itself, it’s crucial to obtain data that exists outside of system records – let’s call this CX-enhancement data. In other words, we model, we mine and then we enhance.
Defining and merging CX and process data
What’s CX-enhancement data? We need to encode the definitions of ‘good’ and ‘bad’ customer experiences for each identified moment of truth in the form of logic checks. This isn’t as simple as it sounds; you need to go the extra mile to seek out these CX metrics above and beyond ‘basic’ process data.
In a fraud reporting example, let’s say customers provide bad feedback when:
- No initial response within one hour.
- No resolution within one business day.
- No refund (if needed) issued within three business days.
We need to encode these expectations into our process mining analytical model: were these expectations met?
The model might look like this:
Activity | Time (hours) | CX score |
Initial response | 0-1 / 1-3 / 3-5/ 5-8 / 8+ | 5 / 4 / 3 / 2 / 1 |
Incident resolution | 0-8 / 8-12 / 12-15 / 15+ | 5 / 4 / 3 / 1 |
Refund issued | 0-72 / 72-96 / 96+ | 5 / 3 / 1 |
With these rules incorporated into our data model, we have made the implicit explicit. In this example, it’s intuitive that taking longer means a worse experience but this is now scored and logged in the data. We’ve created a dataset of moments of truth overlaid onto the process execution record. (If needed, we could ‘weight’ these CX scores to reflect moments of truth that are more significant than others.)
We can now analyse how every execution of the process measured up against what we know about customers’ expectations. CX superpower unlocked!
CX analysis with process mining
Now that we’ve created our process dataset with CX metrics, we can start to generate insights. Start with an exploratory analysis to review the execution record. Using the CX scores that we built into our model, we can create cohorts of cases.
Our goal at this stage is to generate CX-SLAs: via root-cause analysis, when do we start to observe cases gaining the attributes that suggest a poor experience is becoming the likely outcome? We can then flag cases where the calculated ‘CX score’ is falling below expectations based on the stage of the process.
Specifically, the analysis can provide operational teams guidance on ‘remediation windows.’ This window is the time between the case starting to show underperformance and reaching a moment of truth; put simply, there’s still time to take heat out of the fire.
In our analysis, we can flag cases that are in the remediation window and alert operational teams to proactively escalate the case (before the customer does) and avert the moment of truth becoming a moment of pain. Process mining is now actively contributing to achieving the bank’s CX goals, but we’re not done yet.
Proving the value of process mining
We can also generate specific improvement ideas and quantify the expected impact. With the analysis model, we can create reports on certain case cohorts based on the generated CX score:
- Did-not-breach.
- Trended-saved.
- Trended-breached.
- Immediate-breach.
With these cohorts, we can then dig deeper with further analysis and generate recommendations to improve the process. Some CX improvement ideas include:
- Are there specific case types that tend to breach? Can we provide additional training upstream to avoid this?
- Do cases with a poor CX score need more help? Can we recommend a team capacity shift to avoid underperforming activities?
- Can we locate best practice in cases that sail through our CX score checks? Can we share this with other execution teams?
This is all well and good; process mining is now (hopefully) a useful tool in delivering the strategic goal of CX improvement. However, in financial services especially, we’re likely to be under pressure to demonstrate the value creation of process mining in financial terms.
We might have helped operational teams focus on critical CX inflection points, but can we quantify the value of this? Once again, bring your CX-orientated stakeholders together and – downer alert – talk about the cost of underperformance.
We can then log the ‘cost avoidance’ of when we avert closed accounts, escalated complaints and bad reviews in order to show the value of detection, intervention and improvement efforts. In addition, we can use the model we’ve built not just to show the impact of change over time, but to drive change. With our findings, we can recommend alterations to the relevant process and change the documented ‘live’ process. In this way, using the power of process mining within a full transformation suite, we have the means to continuously improve customer experiences, and then quantify those improvements.
CX improvement, like process mining, is a team effort
In each step of this approach, it’s clear that cross-functional engagement is critical to transformation efforts. Not just for creating a high impact process mining analysis, but also for achieving verifiable customer experience improvement. To no-one’s surprise, it turns out strategic goals are hard to attain, and collaboration is a precondition to success.
Where process mining can contribute to customer experience goals in banking is to paint the full picture of the customer’s perspective of actions, going above and beyond reporting the process execution record. We can incorporate existing knowledge of how customers’ experience process outputs into the dataset, and this provides a case-by-case record of how the average customer would react to performance.
The key is to engage the right teams across the bank to obtain critical CX datapoints. It’s entirely possible to directly tie your process mining analysis into meeting CX goals. Without this CX data, don’t expect process performance metrics alone to tell you how customers experience their banking services.
Process mining, we’ve seen, can untangle CX knots and get financial services closer to that Amazon experience that customers demand.